When OpenAI unveiled o1[1] it claimed that its latest large language model (LLM) showed new levels of AI capability. Today, the excitement has largely receded, and the capabilities of this model have been recaptured by open source [2]. For a moment, there was almost a bitter-sweet moment when it almost seemed as if the model had caught up with human capabilities. Beyond marketing, o1 prompted again a discussion about how advanced LLMs really are, how well they capture human abilities, and what it takes to get to an artificial intelligence with the same cognitive capabilities as us.
In this article, we will try to answer the following questions:
- What are the current limits of AI?
- Is the LLM the one that would lead us to human-level AI?
- What do we need for the AGI?
Artificial intelligence is transforming our world, shaping how we live and work. Understanding how it works and its implications has never been more crucial. If you’re looking for simple, clear explanations of complex AI topics, you’re in the right place. Hit Follow or subscribe for free to stay updated with my latest stories and insights.
The limits of a God
The LLM revolution led to speculation that we were close to developing artificial general intelligence (AGI). The arrival of ChatGPT was an ecstatic moment when one could talk to a chatbot with capabilities never seen before, almost as if it were another human being. Then the awe faded away. Until 2022, though, the general public had never asked: is artificial intelligence at the cognitive level of a human being?
This is because the previous models had yes superhuman capabilities but only for specialized applications. For example, AlphaGo [10] had been able to defeat human champions with relative ease, but no one thought that what makes us human was knowing how to play Go. In 2022, though, DALL-E and ChatGPT showed abilities that we generally associate exclusively with humans: creating art and being able to write.
LLMs are not only good at writing but show a range of skills as wide and flexible as ours. In a short time, they have shown that they can pass exams that were usually reserved for humans. This has led to uncanny valley in discussing with them, and to the fear that they may soon replace us in our work.
But do LLMs really have cognitive abilities similar to or superior to humans?
Reasoning and creativity are two abilities that are generally attributed only to humans. Both of these capabilities are difficult to define (and it is difficult to find an unambiguous definition and how to measure them). We have already discussed in a previous article the alleged limitations of LLMs in reasoning. Recent studies rule out that an LLM is truly capable of reasoning. In summary, an LLM uses its gigantic memory to be able to find patterns to answer a question. If it can’t find patterns it is unable to solve a problem.
In addition, recent studies show that an LLM uses a bag of heuristics to solve mathematical calculations. In other words, it uses a set of rules to be able to answer a large number of cases [3]. This is generally enough to answer most of the problems. An LLM either has seen similar patterns in its huge training or can use one of the heuristics. This does not mean real reasoning.
Creativity in writing was recently questioned by a recently published article [4]. Until now, we have not been able to match the LLM’s generated text with what is on the Internet. This makes it difficult to estimate if LLMs are creative or not. New methods allow us to conduct this analysis [4]. The authors clearly show that an LLM is not creative, it is simply text learned during training and regurgitated on demand. In a fair comparison, we see that humans are much more creative than LLMs. According to them, the generated text that seems original comes from private data used in training that we cannot analyze. Also, being a stochastic parrot, the text produced is not exactly the same but with some slight variation.

These results clearly show that LLMs are capable of neither reason nor creativity. LLMs are impressive in finding information in the huge pre-training corpus and responding with this knowledge to a user’s question. However, LLMs are not capable of using this knowledge, recombining it, or creating something new.
The Savant Syndrome: Is Pattern Recognition Equivalent to Intelligence?
Exploring the limits of artificial intelligence: why mastering patterns may not equal genuine reasoning
LLMs and the Student Dilemma: Learning to Solve or Learning to Remember?
Investigating Whether Large Language Models Rely on Genuine Understanding or Clever Heuristics in Arithmetic Reasoning
It is the LLM that will bring us AGI?

No religion needs facts; they all just need miracles and commandments. When the scaling law was published in 2020, many researchers saw both a miracle and a commandment [5]. By increasing the number of parameters, text, and computation, the loss could be linearly decreased and predicted. From there the way was marked and scaling the models became a religion. The second miracle was emergent properties. For many, it meant that we just had to scale the model. Reasoning and creativity in short would appear on their own at some point in scaling.
Not all religions last forever. Faith in the scaling law has begun to creak in the past year. First, emergent properties may not be a real phenomenon but a measurement error. Second, the models do not scale as well as predicted (or at least LLMs are not as powerful as the scaling law predicted). One correction to the dogma was: that there is not just one scaling law, but at least three. For some researchers you have to scale pretraining, scale post-training (alignment, fine-tuning, or any other post-process), and the last scaling law: inference time compute [6].
Sam Altman had vehemently defended the parameter race (after all, this scaling law was a product of OpenAI) but now he does not seem so convinced either:
“When we started the core beliefs were that deep learning works and it gets better with scale… predictably… A religious level belief … was…. that that wasnt’t gotten to stop. .. Then we got the scaling results … At some point you have to just look at the scaling laws and say we’re going to keep doing this… There was something really fundamental going on. We had discovered a new square in the periodic table.” — source
The problem is that the scaling law is not a physical law (however much it has been passed off as such), but a general recipe that states: for most cases, more parameters and more training will lead to better results (lower loss). Loss is not an indication of intelligence, and extrapolating from loss the concept of intelligence is wrong.
In addition, the new scaling law of inference time is not reliable. Performance improves with more steps but after about 20 steps it begins to degrade rapidly. In addition, ChatGPT-4o1 performs better than 4o for only a few cases, showing that this increased thinking time is useful for narrow cases (where reliable synthetic data can be created) and not for open-ended problems.
Another element of concern is that the performance of LLMs is no longer improving exponentially. Ilya Sutskever stated that they are coming to a plateau and that “the 2010s were the age of scaling, now we’re back in the age of wonder and discovery once again.”
This was, however, imaginable. Even if we could build an infinitely large model, we do not have enough quality text to train it [7]:
We find that the total effective stock of human-generated public text data is on the order of 300 trillion tokens, with a 90% confidence interval of 100T to 1000T. This estimate includes only data that is sufficiently high-quality to be used for training, and accounts for the possibility of training models for multiple epochs. — source
The problem is that a model learns only from texts, and if there are no quality texts, it cannot be trained. Quality matters more than just scraping all the possible text. In fact, training on synthetic data is a kind of “knowledge distillation” and can lead to model collapse [16]. Altman states that Orion (which can be considered GPT-5) performs better than previous models but not as much as hoped (e.g., nothing comparable to what we saw between GPT-3 and GPT-4)
LLMs will not magically bring AGI by simply scaling them, that is now well established. The transformer has limitations, it is an exceptional architecture but has generalization limitations. We are simply reaching the limits of a technology that was designed to translate better [8] and luckily has proven to be much more flexible than expected.
A Requiem for the Transformer?
Will be the transformer the model leading us to artificial general intelligence? Or will be replaced?
Can Generative AI Lead to AI Collapse?
AI eating its own tail: the risk of model collapse in generative systems
How to get the AGI?
“With an advanced world model, an AI could develop a personal understanding of whatever scenario it’s placed in, and start to reason out possible solutions.” — Mashrabov, source
The inspiration for having AGI is the human brain. Mostly still some aspects of human cognition are elusive. For some, one of the necessary elements for AGI is the evolution of a ‘world model’. In other words, the human brain learns a representation of the external environment. This representation is used to imagine possible actions or consequences of actions. This model would also be used to generalize tasks we have learned in one domain and apply them to another.
Some researchers claim that LLMs have learned a rudimentary model of the world. For example, in this paper, the authors show that LLMs during training form spatial (and temporal) word models and that these can then be extracted and studied [11].
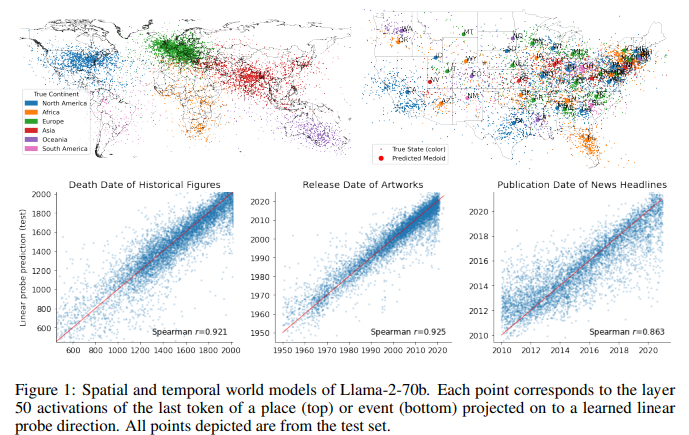
Other elements are also indicating the appearance of an internal representation of the world. Models’ internal representations of color words are similar to facts about human color perception, the ability to make inferences about the beliefs of an author of a document, internally representing the spatial layout of the setting of a story, and the fact that they pass different benchmarks based on commonsense [14].
Other researchers show [12–13] that models trained on transcripts of games like chess or like Othello learn a representation of the world that can then be used to conduct predictions about moves. These moves would be legal and the model would use this representation to estimate the strength of its opponent.